Explore Features and Capabilities of ML Services
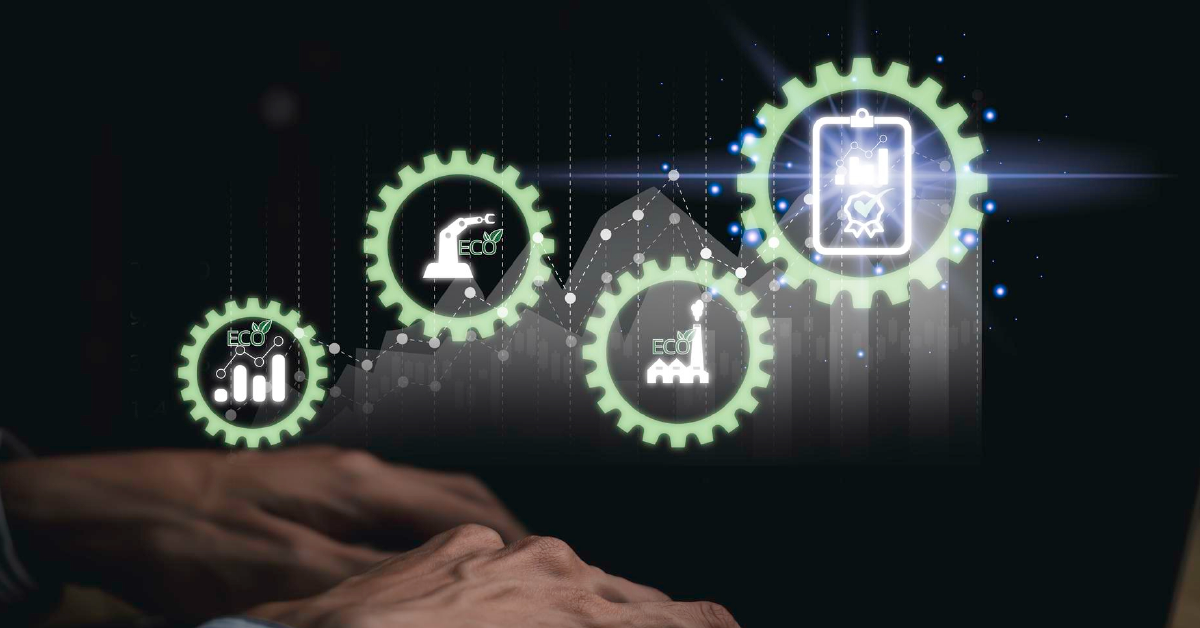
4 min read
Building, training, deploying, and managing customized learning models are made simpler by Machine Learning services. These services offer data science skills through in-database machine learning and direct access to cleaned data, or they do so with the aid of favorite open source libraries and tools. Supporting machine learning services offer greater access to virtual computers or more automated data labelling.
Machine Learning as a Service (MLaaS)
Machine-learning technologies are made available as a part of cloud computing services. The phrase “machine learning as a service” (MLaaS) refers to a collection of these services. Data visualization, APIs, face recognition, Natural language processing (NLP), predictive analytics, and deep learning. These are among the capabilities offered by these services from suppliers. The actual calculation is handled by the provider’s data centers.
The appeal of these services is that, like any other cloud service, clients can get started using machine learning right away without having to set up servers or install software. Learning tools are included in these services, which are provided by numerous cloud providers like Microsoft, Amazon, and IBM. A limited trial of MLaaS is frequently provided so that developers can test it out before committing to a platform and become familiar with it.
Enabling Businesses to Benefit from ML Services
ML can assure exceptional client experience while also enhancing customer loyalty. This is accomplished by leveraging past call records to analyze customer behavior. Then use that data to choose the most qualified customer service professional to handle the client’s request. By doing this, the price and effort spent managing client relationships are significantly reduced. Large companies employ predictive algorithms to provide their customers choices for things they will like.
Important Features of Machine Learning
One of the most significant technological developments in recent years, machine learning has had a profound impact on a variety of fields and applications. Its primary attributes are:
Predictive modelling: Machine learning algorithms use data to build models that predict what will happen in the future. These models can be used, among other things, to assess the risk of loan default or the likelihood that a customer would make a purchase.
Automation: Data patterns are automatically identified by machine learning algorithms, requiring less human involvement and enabling more accurate and effective analysis.
Scalability: Machine learning algorithms are well suited for handling large volumes of data since they are designed to do so. As a result, organizations can use the knowledge gained from such data to inform their actions.
Generalization: Machine learning algorithms are able to identify broad patterns in data that may be applied to the analysis of brand-new, unknown data. The data used to train the model may not be directly pertinent to the task at hand, but they are helpful for predicting the future.
Adaptability: Machine learning algorithms are designed to continuously learn from and adjust as new data becomes available. As a result, they can improve over time, becoming more accurate and effective as more data becomes available.
Machine Learning Capabilities: A Closer Look
Artificial intelligence (AI) is a catch-all phrase for computer software that imitates human brain to carry out challenging tasks and gain knowledge from them. As a branch of artificial intelligence (AI), machine learning (ML) creates flexible models that are capable of a wide range of challenging tasks by using algorithms learned on data.
Pre-built Models and Algorithms
ML services offer a library of pre-built models and algorithms for common use cases like image recognition, natural language processing, and recommendation systems. This accelerates development by allowing developers to leverage these models as a starting point.
Data Integration
Easy integration with data sources is crucial for ML projects. ML services provide connectors and tools to ingest data from various sources, whether it’s structured databases or unstructured data from social media or IoT devices.
- Data storage: ML services often offer data storage solutions that can handle large datasets. Making it easy to store and manage your training and inference data.
- Data preprocessing: Tools for data cleaning, transformation, and feature engineering to prepare data for training.
Auto ML (Automated Machine Learning)
Automated ML capabilities simplify model selection, hyper parameter tuning, and feature engineering. This is especially beneficial for those with limited ML expertise, as it automates many of the complex tasks involved in model development.
Custom Model Training
While pre-built models are useful, ML services also allow you to train custom models using your own data. This is essential for solving unique business challenges that may not have pre-existing solutions.
Real-time Inference
ML services provide APIs and infrastructure for real-time inference, allowing you to make predictions or classifications on new data as it arrives. This is invaluable for applications like fraud detection and recommendation systems.
- Model deployment: Tools for deploying trained models as RESTful APIs or server less functions for real-time inference.
- Batch processing: Capability to perform batch processing on large datasets using your trained models.
Model Monitoring and Management
Ensuring the performance and reliability of ML models over time is critical. ML services offer tools for monitoring model accuracy, drift detection, and automated retraining when necessary.
- Model monitoring: Built-in monitoring for model performance, drift detection, and alerting when issues arise.
- Debugging tools: Debugging and profiling tools to identify and resolve model issues.
Security and Compliance
ML services prioritize security and compliance by providing encryption, access controls, and audit logs. When working with sensitive data, this is essential.
- Access control: Role-based access control (RBAC) to manage who can access and modify ML resources.
- Data encryption: Data encryption in transit and at rest to ensure data security.
- Compliance certifications: Many ML services are compliant with various data protection and industry-specific regulations.
Cost Optimization
Cloud-based ML services offer cost-effective pricing models, including pay-as-you-go options, which allow you to pay only for the resources you use. As a result, there is no longer a requirement for substantial upfront hardware investments.
Cost tracking and management: Tools to monitor and manage ML-related costs to optimize resource usage and control expenses.
Conclusion
Some of the most important characteristics and skills provided by ML services are highlighted here. The best ML service to use will rely on your unique requirements, financial constraints, and level of knowledge with the cloud provider’s platform. Machine Learning (ML) services are cloud-based platforms offered by a number of cloud providers. It can make machine learning models easier to develop, train, deploy, and administer for both individuals and organizations. Without having to spend a lot of money on infrastructure or subject-matter expertise. To make the ML workflow simpler, these platforms offer a wide range of tools and features.
Published: September 14th, 2023