Advancements in Modern Computer Vision Algorithms
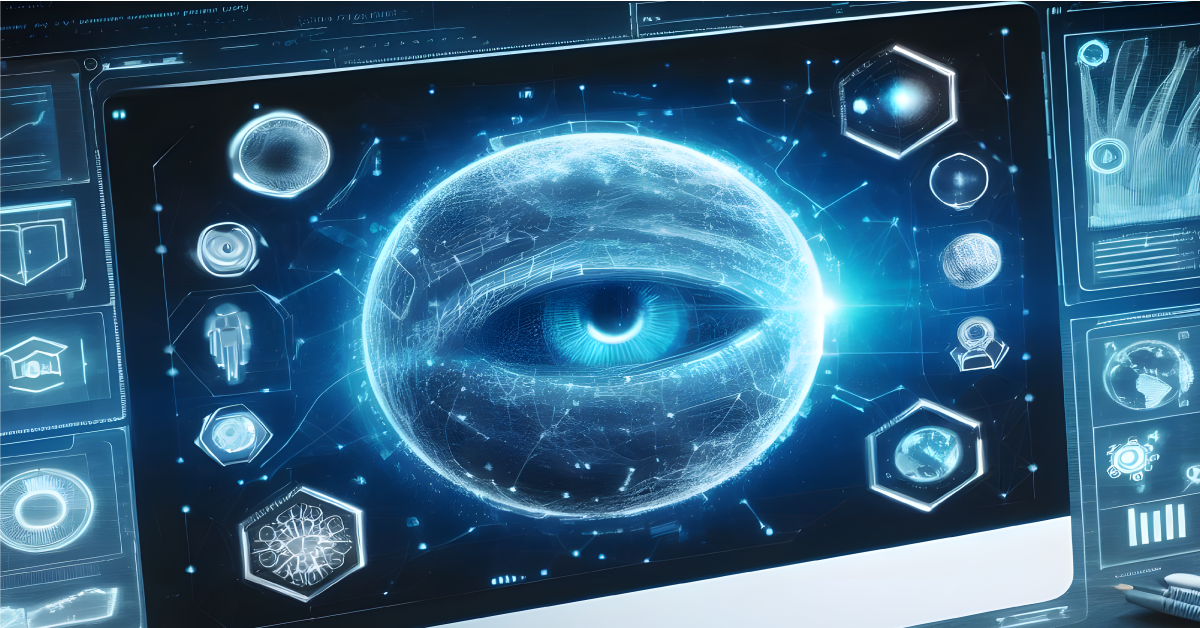
6 min read
Have you ever wondered how computers can “see” and interpret the world around them? With advancements in modern computer vision algorithms, machines can recognize objects, understand gestures, and even drive cars autonomously. We are getting into the latest innovations that are reshaping global industries and setting the way for computer vision in this blog article. Join us as we explore the exciting world of AI-powered visual perception!
Computer vision lies at the heart of artificial intelligence, enabling machines to unlock and make sense of the visual world around us. Its importance cannot be excessive. Computer vision is reshaping our interaction with technology with applications ranging from autonomous vehicles traversing bustling streets to algorithms that diagnose diseases from medical imagery.
Advancement of Computer Vision Algorithms:
The journey of computer vision algorithms has been marked by remarkable evolution—from rudimentary pattern recognition based on simple features to the complex, layered understanding enabled by today’s deep learning models. This evolution reflects our growing ambition to create machines that see as humans do, or perhaps even better.
Traditional Methods vs. Modern Deep Learning Approaches:
The journey from traditional methods to modern deep learning in computer vision showcases a remarkable transformation in our pursuit of creating machines capable of seeing and understanding our world just like we do. This shift highlights technological progress and a deepened understanding of how vision works mechanically and contextually. Paving the way for innovations that bring us closer to machines that perceive the world with the nuance and depth akin to human sight. Initially, Visual perception technology systems were built upon hand-crafted features—meticulously designed algorithms that attempted to imitate the human ability to recognize patterns and shapes. These traditional methods, including edge detection, color analysis, and template matching, were invented in the field, enabling the first steps toward automated image understanding. However, they were often rigid, requiring extensive manual tuning for each new application. And struggled with the complexity and variability inherent in real-world visual data.
Here comes deep learning, a branch of machine learning that processes data using multi-layered neural networks. This approach has fundamentally transformed computer vision. Unlike their predecessors, deep learning models do not rely on human-engineered features. Instead, they automatically discover the representations for feature detection or classification directly from raw images. This shift from manual feature design to automatic feature learning has opened up new boundaries for accuracy and adaptability in computer vision tasks.
Modern Method in Deep Learning:
Its ability to handle various jobs with previously unheard-of efficiency shows its influence on computer vision. For instance, deep learning models can accurately categorize images among thousands of categories in image classification, from identifying species of plants to diagnosing medical images. In object detection, these models go further by recognizing what objects are present in an image. And pinpointing their locations with bounding boxes. Furthermore, deep learning enables complex applications like semantic segmentation, where each pixel in a picture is classified, allowing for detailed scene understanding that is essential for autonomous vehicles and augmented reality systems.
The revolution brought about by deep learning in computer vision is not just about improving accuracy. It has also significantly increased the scalability of computer vision applications. With the ability to learn from large datasets, these models continually improve as more data becomes available. This scalability, coupled with ongoing advancements in computational power and algorithmic efficiency, means that deep learning approaches can increasingly tackle more complex, nuanced visual understanding tasks that were once beyond reach.
Advances in Computer Vision:
Recent years have witnessed exponential growth in computer vision capabilities. Advances in neural network architectures, like convolutional neural networks (CNNs), have paved the way for achievements in real-time image processing, advanced facial recognition, and semantic image segmentation—each leap forward bringing us closer to seamless human-machine interfaces.
Computer vision technology has experienced a meteoric rise in advancement, particularly over the last decade, driven by significant advances in artificial intelligence (AI) and machine learning (ML) technologies. These advancements have dramatically expanded the capabilities of computer vision systems, enabling them to perform tasks with a level of accuracy and effectiveness that was once the stuff of science fiction.
-
Innovative Neural Network Architectures:
Central to these advancements are innovations in neural network architectures, particularly Convolutional Neural Networks (CNNs). CNNs are designed to mechanically and adaptively learn 3-D hierarchies of features from images. This capability has transformed how computers interpret visual information. And making them incredibly effective for tasks involving image recognition, object detection, and classification.
-
Real-time Image Processing:
One of the most impactful advancements has been the ability to process and analyze images in real-time. This development is important for applications requiring immediate feedback, such as autonomous vehicle navigation, where split-second decisions based on visual inputs can be the difference between safety and collision. Real-time image processing has also heightened user experiences in interactive applications, like video games and live video enhancements, by allowing instant adjustments and responses to visual data.
-
Advanced Facial Recognition:
Facial recognition technology has advanced dramatically, moving from simple pattern recognition to advanced systems capable of accurately identifying individuals among millions. This technology now supports many applications, from security systems granting access based on facial features to automatically tagging friends in social media photos. The evolution of facial recognition reflects broader trends in computer vision. Systems recognize, understand, and interpret visual data with nuanced precision.
-
Semantic Image Segmentation:
Semantic image segmentation represents another frontier where computer vision has made significant progress. This technique involves classifying each pixel in an image into a category, allowing the system to understand the picture at the pixel level. This depth of understanding is important for detailed scene analysis required in medical imaging. Distinguishing between healthy and diseased tissue can aid in diagnosis and treatment plans. And in autonomous driving systems, distinguishing between a pedestrian and a lamppost can be vital.
-
Expanding Human-Machine Interfaces:
Each of these computer vision advancements contributes to the development of more seamless and intuitive human-machine interfaces. By enabling machines to see and interpret the world more like humans do, we are opening up new possibilities for interaction. Whether through gesture recognition that allows users to control devices with a wave of a hand or through augmented reality systems that overlay digital information onto the physical world. And the goal is to create interfaces that are as natural and effortless as possible.
-
Application Areas of Modern Computer Vision Algorithms:
The applications of modern computer vision are diverse and expanding:
Healthcare: Enhancing diagnostic accuracy and patient care through automated image analysis.
Security: Transforming surveillance systems with real-time threat detection.
Retail: Altering customer experiences through smart checkout systems and inventory management.
Agriculture: Optimizing crop yield predictions and monitoring plant health via aerial imagery.
Challenges and Limitations of Current Techniques:
Despite significant strides, challenges persist in data-centric computer vision research. Issues of data bias, computational resource requirements, and the ongoing quest for generalizability across diverse visual contexts underscore the complex nature of teaching machines to see through a data-centric approach.
The Future Holds for Potential Developments in the Field:
Looking forward, the horizon of Visual perception technology brims with potential. Innovations such as few-shot learning, which aims to recognize objects from very few examples, and integrating Visual perception technology with other AI domains promise to expand the achievable boundaries further.
Impact of Modern Computer Vision Algorithms on Society:
The societal impact of modern computer vision algorithms is profound, touching every aspect of our lives—from enhancing public safety and healthcare outcomes to driving the future of entertainment and personal technology. As these algorithms become more integrated into daily life, their role in shaping future societal structures continues to grow.
Conclusion:
The advancements in modern computer vision algorithms represent a monumental leap forward in how machines understand the world. And opening up possibilities that were once deemed the field of science fiction. From the evolution of simple pattern recognition to the advanced understanding enabled by deep learning models. We have seen visual perception technology enhance everything from healthcare diagnostics to autonomous vehicle navigation.
These technologies improve the accuracy and efficiency of tasks and revolutionize user experiences and interfaces. And making interactions with machines more natural and intuitive. As we continue to push the boundaries of what’s possible, integrating these advancements across various sectors promises to transform our society profoundly. At Pranathi Software Services, we are excited to be at the lead of these innovations, leveraging advanced computer vision algorithms to deliver solutions that drive progress and reshape industries. The future of Visual perception technology is not just about enhancing the way machines see. It’s about envisioning a world where technology and human insight merge seamlessly to create a smarter, safer, and more connected world.
FAQ:
1. What Is Computer Vision?
Computer vision is a field of artificial intelligence that enables machines to interpret. And understand visual information from the world around them, similar to how humans use their eyes to see.
2. How Have Neural Networks Impacted Computer Vision?
Neural networks, particularly Convolutional Neural Networks (CNNs), have revolutionized Visual perception technology by enabling more accurate and efficient image recognition, object detection, and classification, significantly advancing the field.
3. What Are Some Real-world Applications of Computer Vision?
Computer vision applications span various sectors, including autonomous driving, facial recognition for security, medical image analysis for healthcare, augmented reality for interactive experiences, and more.
4. What Makes Deep Learning Different from Traditional Computer Vision Techniques?
Deep learning automates the feature extraction process, learning directly from data, which contrasts with traditional methods that rely on manually defined features and rules. This difference allows for greater accuracy and adaptability in visual tasks.
5.Can Computer Vision Algorithms Process Images in real-time?
Yes, advancements in computational power and algorithm efficiency have enabled computer vision algorithms to process. And analyze images in real time, which is crucial for applications like autonomous driving and interactive gaming.
Published: April 30th, 2024