From Text to Insights: Demystifying NLP in the BFSI Sector
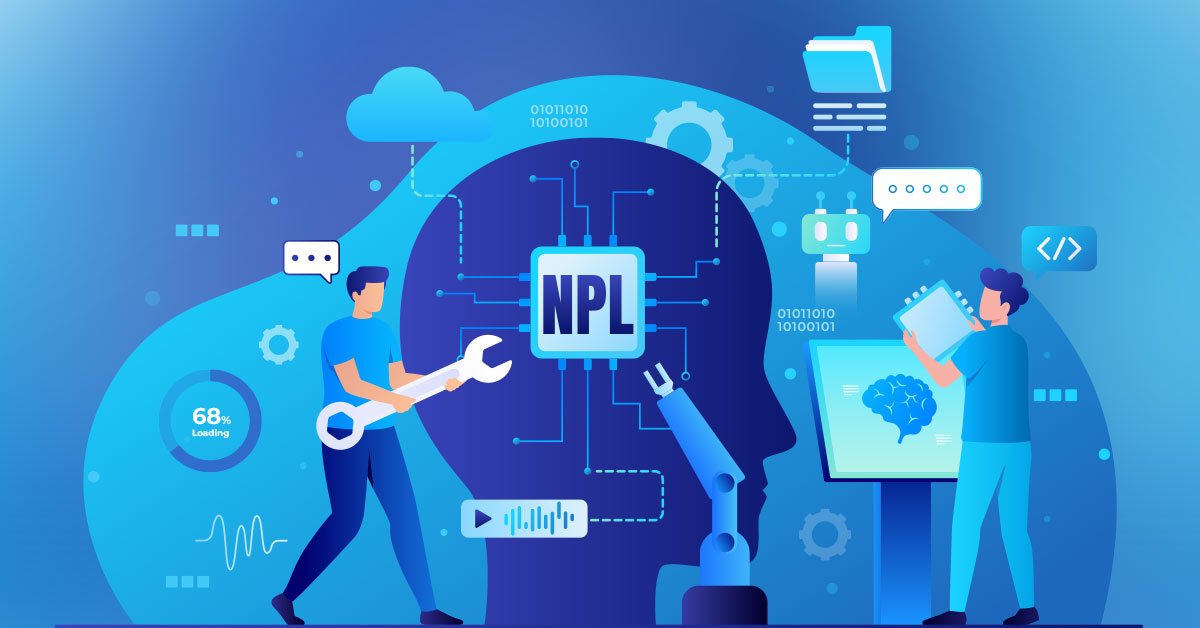
6 min read
The banking, financial services, and insurance (BFSI) sector generates enormous amounts of text data every day. Unstructured material is abundant in this industry, ranging from market reports and financial records to customer comments and service queries. Making sense of the data is essential to enhancing customer experience, lowering risk, and arriving at wise business decisions. A key component of artificial intelligence (AI) is the science of natural language processing (NLP), which transforms this text data into useful insights. We will go over NLP in the BFSI industry in this blog.
Understanding Natural Language Processing is important for the Banking, Financial Services, and Insurance (BFSI) industry since it helps to extract value from various types of unstructured text data. This data may be debilitating in terms of amount and complexity. It comes from a variety of sources, including emails, social media posts, legal documents, and market news. This abundance of textual data can be processed and analyzed, and valuable insights may be extracted from it with ease by the BFSI sector through language processing approaches.
NLP algorithms enable BFSI firms to carry out a variety of tasks, including topic modelling, text categorization into themes, summarization, reducing large amounts of content, sentiment analysis, evaluating customer feedback, entity recognition, and finding key entities in documents. By improving decision-making procedures and enabling a more thorough comprehension of consumer attitudes, market trends, and regulatory environments, the BFSI industry uses natural processing language. It helps the industry remain flexible and efficient in the face of changing financial conditions.
Application of NLP in the BFSI Sector
In BFSI industry, it has a wide range of uses that are revolutionizing how businesses manage and extract value from unstructured text data. Important uses of NLP in BFSI include the following:
-
Sentiment Analysis:
NLP data analytics algorithms analyze sentiment on textual data, such as reviews from customers, social media posts, and other textual data. It assists financial firms in managing reputational risks, identifying possible problems, and gauging consumer happiness.
-
Entity Recognition
Names, companies, and financial words are examples of specific entities that can be recognized and extracted from unstructured text using language processing. It facilitates the extraction of information from emails, news stories, and documents.
-
Customer Service Chatbots
Natural language processing engines are the driving force behind intelligent chatbots that enhance customer interactions. These conversational agents possess the ability to comprehend and react to client inquiries, offering instant support and enhancing the entire customer experience.
-
Fraud Detection
Patterns suggestive of fraudulent activity can be found in textual data by using natural processing language. It enhances fraud detection systems by providing context and subtleties in textual input.
-
Regulatory Compliance
BFSI companies use NLP to better effectively traverse regulatory environments. These algorithms help ensure compliance with intricate legislation by reading and extracting pertinent information from legal papers.
-
Market Analysis
Financial reports, market news, and other textual sources may all be categorized and analyzed. It helps with following market patterns, forecasting changes, and making wise investment choices.
-
Document Summarization
Text analysis algorithms are capable of summarizing extensive legal papers, reports, or contracts. It improves the effectiveness of document review procedures and enables experts to swiftly assimilate vital information.
-
Personalized Financial Advice
By analyzing client communications, processing language can be used to customize financial advice according to each person’s preferences, risk tolerance, and financial objectives.
I. The Role of NLP in BFSI
-
Data Overload in BFSI
The industry that produces the most textual data, Banking, Financial Services, and Insurance (BFSI), is at the forefront of information generation. This data stream comes from a wide range of sources, including legal records, customer chats, and intricate financial reports. For industry professionals looking to extract useful insights, the sheer volume of textual information created on a daily basis is a significant problem.
The BFSI industry generates a vast amount of unstructured data alone from client interactions. Email correspondence, chat logs, and customer reviews are just a few examples of textual data that can provide a plethora of insights that, when used wisely, can revolutionize marketing plans, customer support, and general business operations.
A constant flow of regulatory paperwork is also a result of the strict regulatory frameworks that bind the BFSI sector. These documents significantly expand the industry’s textual data pool and are often replete with complex jargon and ever-changing compliance regulations. Institutions find it difficult to manually process and extract meaningful insights from such large datasets due to the additional complexity created by financial reports, market assessments, and economic forecasts.
II. Challenges Addressed by NLP
-
Manual Analysis Limitations
In the past, the BFSI sector mostly depended on human analysis to extract the information concealed in textual data. But with all the data and its complexity, this method is becoming more and more insufficient. Manual analysis takes a lot of time, is leads to mistakes, and slows down the extraction of insights. Automation of the analysis process improves accuracy and efficiency considerably. Natural language processing provides an answer with its large-scale natural language understanding and processing capabilities.
-
Information Overload
The enormous volume of textual data generated is directly responsible for the information overload in the BFSI industry. It might be difficult to sift through this information flood and find pertinent data, new trends, and possible dangers. These algorithms are excellent at identifying patterns, sifting through large datasets, and emphasizing important information. It facilitates effective decision-making and assurances that the deluge of irrelevant data does not obscure important insights.
-
Need for Real-Time Understanding
Real-time comprehension of textual data is essential in the quick-paced world of finance, where market conditions might change at any time. The flexibility needed to react quickly to changes in the market or new threats is not provided by manual processing. Real-time natural language processing provides banking and financial services (BFSI) professionals with timely insights that enable them to react quickly to changing market conditions and make well-informed decisions.
Read more articles: Natural Language Processing for Better Website Interactions
III. Future Trends: Navigating the Evolving Landscape of NLP in the BFSI Sector
Natural language processing will play an increasingly important role as the BFSI industry continues to change. A number of developments indicate how technology is changing the sector and how it can further transform how financial institutions get insights from textual data.
-
Advanced Sentiment Analysis
It is expected that sentiment analysis using NLP services will become increasingly sophisticated and context-aware in the future. Traditional sentiment analysis divides text into three categories: positive, negative, and neutral. In the future, more sophisticated sentiment analysis models are expected. These models will be capable of recognizing minute details and identifying sentiments impacted by local, cultural, or industry-specific settings. Decision-making procedures would be improved as a result of BFSI workers’ increased understanding of consumer and market sentiments.
-
Explainable AI in Regulatory Compliance
As regulatory requirements get more complex, AI models, including natural language processing and AI, that offer clear justifications for their decisions are becoming more and more necessary. Explainable AI will be essential for tackling issues with regulatory compliance. To ensure compliance and build trust in the decision-making process, institutions will increasingly rely on processing language models. These models should be capable of producing understandable, concise explanations for the insights they extract from regulatory texts.
-
Conversational AI in Customer Service
Conversational AI integration in customer support is about to take off. Natural language processing will enable chatbots and virtual assistants to become more intelligent and capable of engaging in dynamic, context-aware conversations. These AI-powered interfaces will improve client interactions while streamlining repetitive banking operations and providing a smooth, customized experience.
-
Multilingual NLP for Global Operations
The need for multilingual language processing skills is expected to grow in the increasingly interconnected global economy. Businesses within BFSI that operate in a variety of linguistic environments will make use of NLP models that include multilingual understanding and processing skills. This pattern will make it easier to analyze text data accurately and meaningfully from various geographical locations. It will allow institutions to adjust their tactics to suit regional quirks effectively.
-
Hybrid Models for Enhanced Risk Management
In the future, NLP for risk management will use hybrid models. These models will provide a thorough method of risk identification and mitigation. They achieve this by combining the advantages of machine-learning algorithms with rule-based systems. Financial institutions will be able to handle complicated risk scenarios more accurately and effectively through hybrid NLP models.
-
Real-Time Fraud Detection with Continuous Learning
Continuous learning will be included in NLP systems for fraud detection in order to counter developing threats. By adapting and learning from new data patterns, real-time fraud detection systems will remain ahead of developing fraud strategies. This proactive approach will better protect financial institutions and their clients against emerging cyber risks.
-
Semantic Search for Enhanced Information Retrieval
The development of semantic search will revolutionize the way financial professionals obtain information. Semantic search engines powered by natural processing language will understand the meaning and context of search requests and return more pertinent and accurate results. This pattern will improve BFSI’s information retrieval operations’ effectiveness and speed up decision-making.
Conclusion
Natural language processing (NLP) has had a revolutionary impact on the BFSI industry, as demonstrated by “From Text to Insights”. It has proven to be an effective solution for addressing the issues of manual analysis and real-time understanding in the face of the data flood of customer communications, regulatory documents, and financial reports.
Prospects for Natural processing language in BFSI include conversational interfaces, multilingual capabilities, explainable AI, sophisticated sentiment analysis, and more. The changes above indicate a paradigm shift in the extraction of insights from textual material, providing enhanced efficiency and comprehension.
Financial organizations benefit from the incorporation of language technology by managing risks more effectively, gaining insights from conversations, and improving client relations. The storyline changes from being overtaken by data to being a strategic advantage, placing BFSI at the forefront of innovation in a changing financial environment.
NLP helps BFSI on its path toward a future in which every textual data point can yield revolutionary insights that lead to long-term success. Institutions that adopt information retrievals enter a new era where data is a gateway to unmatched insights rather than just information. It is just the beginning of a story that promises a future in which data will be the driving force behind unheard-of growth through natural language processing.
Let’s begin to explore more about artificial intelligence services.
Published: December 6th, 2023